Telehealth has emerged as a vital channel for health services delivery in the post-COVID era. However, understanding patient telehealth use remains a complex challenge for healthcare providers. At EVERSANA, our experts are leveraging machine learning techniques to predict this effectively, providing valuable insights for healthcare marketing and patient engagement strategies.
Pierantonio Russo, M.D., EVERSANA’s Corporate Chief Medical Officer, and the Data Science team, led by Ramaa Nathan, PhD are at the forefront of this innovative approach. As he describes as the “Hospital Without Walls”, Russo has already predicted how telemedicine will revolutionize care now and in the future. But how can we know how much patients will really use it? And in what types of contexts? Machine learning, a type of AI could be the solution. The term Machine learning coined by Arthur Lee Samuel in 1959 and it is the
“Field of study that gives computers the ability to learn without being explicitly programmed”. In other words, “ML “learns” from datasets, and it predicts outcomes leveraging multiple inputs and patterns relevant to the question to be addressed.”
With a focus on combining real-world claims data, social determinants of health, and network-level telehealth details, EVERSANA has developed robust predictive modeling and clustering techniques to identify patients most likely to utilize telehealth services.
Presenting these findings at the PMSA Annual Conference, March 5-8 in Denver, Colorado.
At the 2024 PMSA Annual Conference, Dr. Russo’s team will showcase their approach in detail, focusing on the design of two types of models, patient proactivity and visit accessibility. What are these different models? Let’s take a look:
- Patient Proactivity: This model is based on a patient’s internal willingness to choose telehealth services. It uses patient-level features such as region, rural vs. urban location type, age, and overall number of visits to identify cohorts of patients who are likely to choose telehealth services over in-office visits.
- Visit Accessibility: This model focuses on a patient’s external access to telehealth services. It uses individual visit-level features like HCP specialty, network telehealth usage, and prescription association to identify visits that are telehealth-accessible to patients.
By combining these two metrics with clustering algorithms, the EVERSANA team generated different telehealth-use personas for several distinct therapeutic areas, and they’ve been able to help predict how many patients may utilize telehealth services in the future, bringing unmatched opportunities for pharmaceutical brands to further the approach of building programs to serve patients.The innovative approach presented by Dr. Russo and his team highlights the power of combining machine learning techniques with real-world data to predict patient behavior in the growing field of telemedicine. This not only provides valuable insights into patient telehealth use but also enables fine-tuned marketing strategies and targeted patient engagement.
“The future of patient care has been transformed through the power of data and analytics leveraging the integration of clinical science, population medicine and health economics with AI and ML delivering unmatched value to brands, patients, and larger communities,” noted Russo.
Interested in learning more about EVERSANA’s Data & Analytics capabilities? Click here.
Author
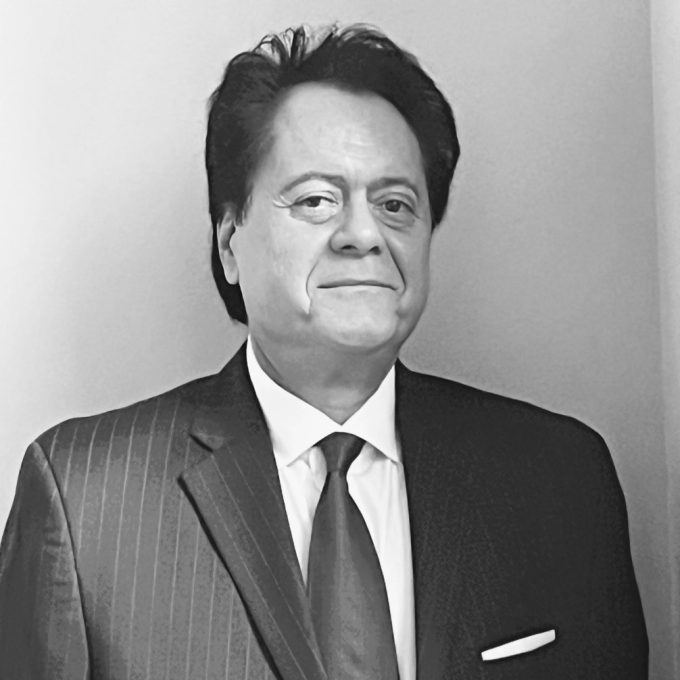
After leaving the Mayo Clinic, from 1988 to 2007, he held academic, clinical and administrative leadership positions as Chief of Cardiac Surgery, Pediatric Cardiac Surgery and Heart Transplantation at several Academic Hospitals in the…